Lab 4: Data engineering pipelines#
# Auto-setup when running on Google Colab
if 'google.colab' in str(get_ipython()):
!pip install openml
# General imports
%matplotlib inline
import numpy as np
import pandas as pd
import matplotlib.pyplot as plt
import openml as oml
import seaborn as sns
Building Pipelines#
In scikit-learn, a
pipeline
combines multiple processing steps in a single estimatorAll but the last step should be transformer (have a
transform
method)The last step can be a transformer too (e.g. Scaler+PCA)
It has a
fit
,predict
, andscore
method, just like any other learning algorithmPipelines are built as a list of steps, which are (name, algorithm) tuples
The name can be anything you want, but can’t contain
'__'
We use
'__'
to refer to the hyperparameters, e.g.svm__C
Let’s build, train, and score a
MinMaxScaler
+LinearSVC
pipeline:
pipe = Pipeline([("scaler", MinMaxScaler()), ("svm", LinearSVC())])
pipe.fit(X_train, y_train).score(X_test, y_test)
from sklearn.pipeline import Pipeline
from sklearn.preprocessing import MinMaxScaler
from sklearn.svm import LinearSVC
from sklearn.model_selection import train_test_split
from sklearn.datasets import load_breast_cancer
cancer = load_breast_cancer()
pipe = Pipeline([("scaler", MinMaxScaler()), ("svm", LinearSVC())])
X_train, X_test, y_train, y_test = train_test_split(cancer.data, cancer.target,
random_state=1)
pipe.fit(X_train, y_train)
print("Test score: {:.2f}".format(pipe.score(X_test, y_test)))
Test score: 0.97
Now with cross-validation:
scores = cross_val_score(pipe, cancer.data, cancer.target)
from sklearn.model_selection import cross_val_score
scores = cross_val_score(pipe, cancer.data, cancer.target)
print("Cross-validation scores: {}".format(scores))
print("Average cross-validation score: {:.2f}".format(scores.mean()))
Cross-validation scores: [0.98245614 0.97368421 0.96491228 0.96491228 0.99115044]
Average cross-validation score: 0.98
We can retrieve the trained SVM by querying the right step indices
pipe.steps[1][1]
pipe.fit(X_train, y_train)
print("SVM component: {}".format(pipe.steps[1][1]))
SVM component: LinearSVC()
Or we can use the
named_steps
dictionary
pipe.named_steps['svm']
print("SVM component: {}".format(pipe.named_steps['svm']f))
SVM component: LinearSVC()
When you don’t need specific names for specific steps, you can use
make_pipeline
Assigns names to steps automatically
pipe_short = make_pipeline(MinMaxScaler(), LinearSVC(C=100))
print("Pipeline steps:\n{}".format(pipe_short.steps))
from sklearn.pipeline import make_pipeline
# abbreviated syntax
pipe_short = make_pipeline(MinMaxScaler(), LinearSVC(C=100))
print("Pipeline steps:\n{}".format(pipe_short.steps))
Pipeline steps:
[('minmaxscaler', MinMaxScaler()), ('linearsvc', LinearSVC(C=100))]
Visualization of a pipeline fit
and predict

Using Pipelines in Grid-searches#
We can use the pipeline as a single estimator in
cross_val_score
orGridSearchCV
To define a grid, refer to the hyperparameters of the steps
Step
svm
, parameterC
becomessvm__C
param_grid = {'svm__C': [0.001, 0.01, 0.1, 1, 10, 100],
'svm__gamma': [0.001, 0.01, 0.1, 1, 10, 100]}
from sklearn import pipeline
from sklearn.svm import SVC
from sklearn.model_selection import GridSearchCV
pipe = pipeline.Pipeline([("scaler", MinMaxScaler()), ("svm", SVC(C=100))])
grid = GridSearchCV(pipe, param_grid=param_grid, cv=5)
grid.fit(X_train, y_train)
print("Best cross-validation accuracy: {:.2f}".format(grid.best_score_))
print("Test set score: {:.2f}".format(grid.score(X_test, y_test)))
print("Best parameters: {}".format(grid.best_params_))
Best cross-validation accuracy: 0.97
Test set score: 0.97
Best parameters: {'svm__C': 10, 'svm__gamma': 1}
When we request the best estimator of the grid search, we’ll get the best pipeline
grid.best_estimator_
print("Best estimator:\n{}".format(grid.best_estimator_))
Best estimator:
Pipeline(steps=[('scaler', MinMaxScaler()), ('svm', SVC(C=10, gamma=1))])
And we can drill down to individual components and their properties
grid.best_estimator_.named_steps["svm"]
# Get the SVM
print("SVM step:\n{}".format(
grid.best_estimator_.named_steps["svm"]))
SVM step:
SVC(C=10, gamma=1)
# Get the SVM dual coefficients (support vector weights)
print("SVM support vector coefficients:\n{}".format(
grid.best_estimator_.named_steps["svm"].dual_coef_))
SVM support vector coefficients:
[[ -1.39188844 -4.06940593 -0.435234 -0.70025696 -5.86542086
-0.41433994 -2.81390656 -10. -10. -3.41806527
-7.90768285 -0.16897821 -4.29887055 -1.13720135 -2.21362118
-0.19026766 -10. -7.12847723 -10. -0.52216852
-3.76624729 -0.01249056 -1.15920579 -10. -0.51299862
-0.71224989 -10. -1.50141938 -10. 10.
1.99516035 0.9094081 0.91913684 2.89650891 0.39896365
10. 9.81123374 0.4124202 10. 10.
10. 5.41518257 0.83036405 2.59337629 1.37050773
10. 0.27947936 1.55478824 6.58895182 1.48679571
10. 1.15559387 0.39055347 2.66341253 1.27687797
0.65127305 1.84096369 2.39518826 2.50425662]]
Grid-searching preprocessing steps and model parameters#
We can use grid search to optimize the hyperparameters of our preprocessing steps and learning algorithms at the same time
Consider the following pipeline:
StandardScaler
, without hyperparametersPolynomialFeatures
, with the max. degree of polynomialsRidge
regression, with L2 regularization parameter alpha
from sklearn.datasets import fetch_california_housing
from sklearn.preprocessing import StandardScaler
from sklearn.linear_model import Ridge
housing = fetch_california_housing()
X_train, X_test, y_train, y_test = train_test_split(housing.data, housing.target,
random_state=0)
from sklearn.preprocessing import PolynomialFeatures
pipe = pipeline.make_pipeline(
StandardScaler(),
PolynomialFeatures(),
Ridge())
We don’t know the optimal polynomial degree or alpha value, so we use a grid search (or random search) to find the optimal values
param_grid = {'polynomialfeatures__degree': [1, 2, 3],
'ridge__alpha': [0.001, 0.01, 0.1, 1, 10, 100]}
grid = GridSearchCV(pipe, param_grid=param_grid, cv=5, n_jobs=1)
grid.fit(X_train, y_train)
param_grid = {'polynomialfeatures__degree': [1, 2, 3],
'ridge__alpha': [0.001, 0.01, 0.1, 1, 10, 100]}
# Note: I had to use n_jobs=1. (n_jobs=-1 stalls on my machine)
grid = GridSearchCV(pipe, param_grid=param_grid, cv=5, n_jobs=1)
grid.fit(X_train, y_train);
Visualing the \(R^2\) results as a heatmap:
import matplotlib.pyplot as plt
plt.matshow(grid.cv_results_['mean_test_score'].reshape(3, -1),
vmin=0, cmap="viridis")
plt.xlabel("ridge__alpha")
plt.ylabel("polynomialfeatures__degree")
plt.xticks(range(len(param_grid['ridge__alpha'])), param_grid['ridge__alpha'])
plt.yticks(range(len(param_grid['polynomialfeatures__degree'])),
param_grid['polynomialfeatures__degree'])
plt.colorbar();
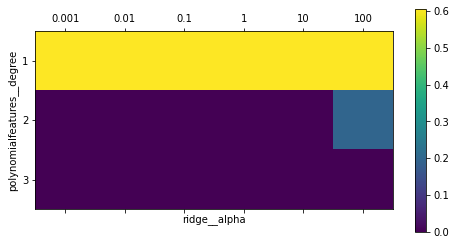
Here, degree-2 polynomials help (but degree-3 ones don’t), and tuning the alpha parameter helps as well.
Not using the polynomial features leads to suboptimal results (see the results for degree 1)
print("Best parameters: {}".format(grid.best_params_))
print("Test-set score: {:.2f}".format(grid.score(X_test, y_test)))
Best parameters: {'polynomialfeatures__degree': 1, 'ridge__alpha': 10}
Test-set score: 0.59
FeatureUnions#
Sometimes you want to apply multiple preprocessing techniques and use the combined produced features
Simply appending the produced features is called a
FeatureJoin
Example: Apply both PCA and feature selection, and run an SVM on both
from sklearn.pipeline import Pipeline, FeatureUnion
from sklearn.svm import SVC
from sklearn.datasets import load_iris
from sklearn.decomposition import PCA
from sklearn.feature_selection import SelectKBest
iris = load_iris()
X, y = iris.data, iris.target
# This dataset is way too high-dimensional. Better do PCA:
pca = PCA(n_components=2)
# Maybe some original features where good, too?
selection = SelectKBest(k=1)
# Build estimator from PCA and Univariate selection:
combined_features = FeatureUnion([("pca", pca), ("univ_select", selection)])
# Use combined features to transform dataset:
X_features = combined_features.fit(X, y).transform(X)
print("Combined space has", X_features.shape[1], "features")
svm = SVC(kernel="linear")
# Do grid search over k, n_components and C:
pipeline = Pipeline([("features", combined_features), ("svm", svm)])
param_grid = dict(features__pca__n_components=[1, 2, 3],
features__univ_select__k=[1, 2],
svm__C=[0.1, 1, 10])
grid_search = GridSearchCV(pipeline, param_grid=param_grid)
grid_search.fit(X, y)
print(grid_search.best_estimator_)
Combined space has 3 features
Pipeline(steps=[('features',
FeatureUnion(transformer_list=[('pca', PCA(n_components=3)),
('univ_select',
SelectKBest(k=1))])),
('svm', SVC(C=10, kernel='linear'))])
ColumnTransformer#
A pipeline applies a transformer on all columns
If your dataset has both numeric and categorical features, you often want to apply different techniques on each
You could manually split up the dataset, and then feature-join the processed features (tedious)
ColumnTransformer
allows you to specify on which columns a preprocessor has to be runEither by specifying the feature names, indices, or a binary mask
You can include multiple transformers in a ColumnTransformer
In the end the results will be feature-joined
Hence, the order of the features will change! The features of the last transformer will be at the end
Each transformer can be a pipeline
Handy if you need to apply multiple preprocessing steps on a set of features
E.g. use a ColumnTransformer with one sub-pipeline for numerical features and one for categorical features.
In the end, the columntransformer can again be included as part of a pipeline
E.g. to add a classfier and include the whole pipeline in a grid search
Example: Handle a dataset (Titanic) with both categorical an numeric features
Numeric features: impute missing values and scale
Categorical features: Impute missing values and apply one-hot-encoding
Finally, run an SVM
from sklearn.compose import ColumnTransformer
from sklearn.datasets import fetch_openml
from sklearn.impute import SimpleImputer
from sklearn.preprocessing import StandardScaler, OneHotEncoder
from sklearn.linear_model import LogisticRegression
from sklearn.model_selection import train_test_split, GridSearchCV
np.random.seed(0)
# Load data from https://www.openml.org/d/40945
X, y = fetch_openml("titanic", version=1, as_frame=True, return_X_y=True)
# Alternatively X and y can be obtained directly from the frame attribute:
# X = titanic.frame.drop('survived', axis=1)
# y = titanic.frame['survived']
# We will train our classifier with the following features:
# Numeric Features:
# - age: float.
# - fare: float.
# Categorical Features:
# - embarked: categories encoded as strings {'C', 'S', 'Q'}.
# - sex: categories encoded as strings {'female', 'male'}.
# - pclass: ordinal integers {1, 2, 3}.
# We create the preprocessing pipelines for both numeric and categorical data.
numeric_features = ['age', 'fare']
numeric_transformer = Pipeline(steps=[
('imputer', SimpleImputer(strategy='median')),
('scaler', StandardScaler())])
categorical_features = ['embarked', 'sex', 'pclass']
categorical_transformer = Pipeline(steps=[
('imputer', SimpleImputer(strategy='constant', fill_value='missing')),
('onehot', OneHotEncoder(handle_unknown='ignore'))])
preprocessor = ColumnTransformer(
transformers=[
('num', numeric_transformer, numeric_features),
('cat', categorical_transformer, categorical_features)])
# Append classifier to preprocessing pipeline.
# Now we have a full prediction pipeline.
clf = Pipeline(steps=[('preprocessor', preprocessor),
('classifier', LogisticRegression())])
X_train, X_test, y_train, y_test = train_test_split(X, y, test_size=0.2)
clf.fit(X_train, y_train)
print("model score: %.3f" % clf.score(X_test, y_test))
model score: 0.790
You can again run optimize any of the hyperparameters (preprocessing-related ones included) in a grid search
param_grid = {
'preprocessor__num__imputer__strategy': ['mean', 'median'],
'classifier__C': [0.1, 1.0, 10, 100],
}
grid_search = GridSearchCV(clf, param_grid, cv=10)
grid_search.fit(X_train, y_train)
print(("best logistic regression from grid search: %.3f"
% grid_search.score(X_test, y_test)))
best logistic regression from grid search: 0.798